AI Readiness Framework Strategy
A comprehensive framework to assess and enhance your organization's readiness for generative AI adoption
Generative AI Readiness Framework
A structured approach to assess and enhance AI readiness for IT services companies looking to harness generative AI.
Artificial intelligence – and specifically generative AI – is transforming how businesses innovate and operate. While 79% of Canadian CEOs plan to adopt AI within the next year, only 1% consider their companies "fully mature" in AI deployment. This gap between ambition and reality is significant: over 80% of AI projects fail or are abandoned before delivering value.
This framework helps organizations self-assess their maturity across four essential pillars, identify gaps, and take actionable steps to become "AI-ready."
79%
of Canadian CEOs Plan to Adopt AI
80%+
AI Projects Fail to Deliver Value
1%
Consider AI Fully Mature
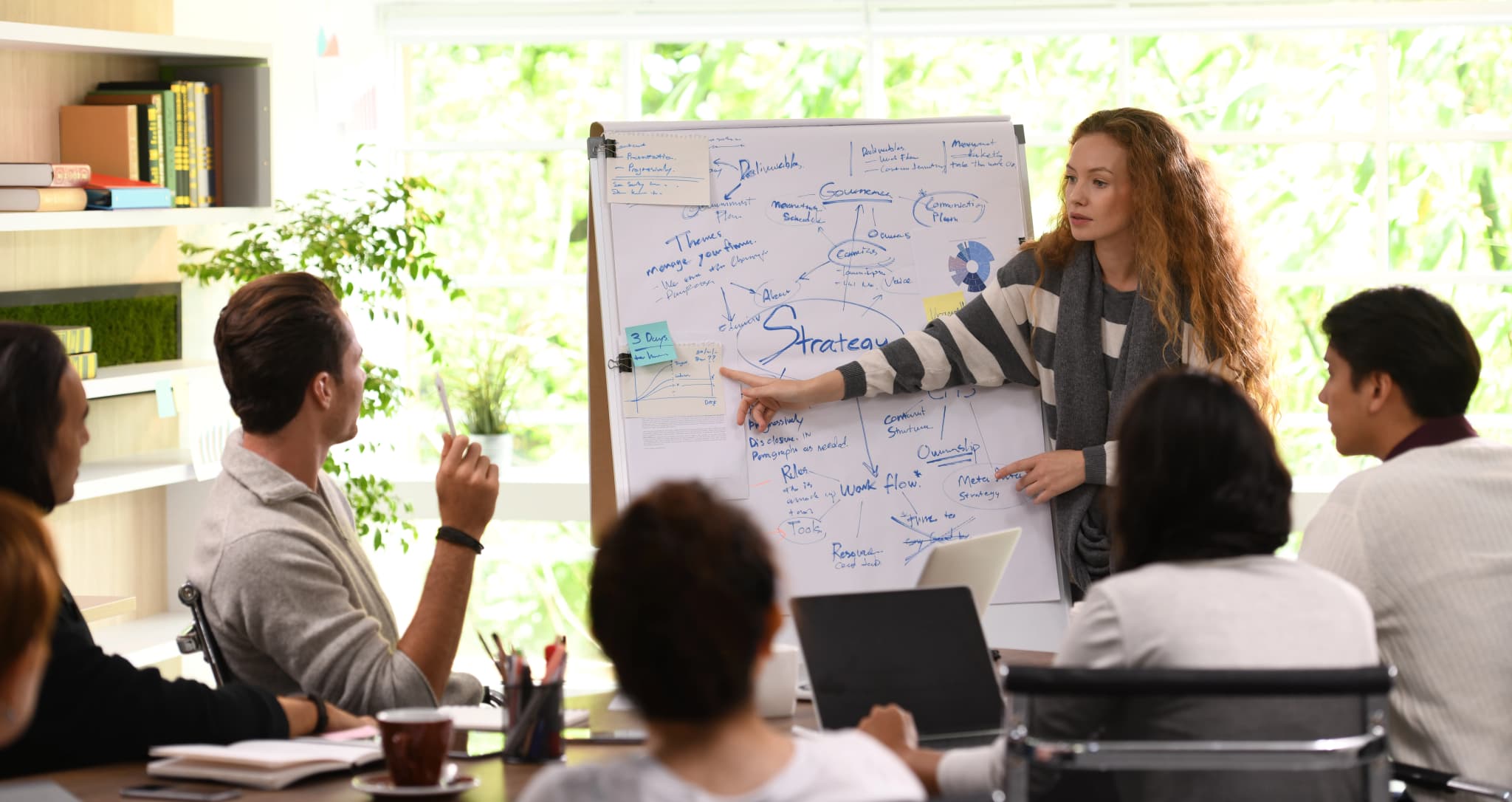
AI Insight
Most organizations fall into the AI readiness gap: high ambition but low implementation maturity.
Why a Readiness Framework Matters
Four Pillars of AI Readiness
A comprehensive assessment across these key dimensions ensures successful AI implementation
Readiness Framework Overview
This framework presents a comprehensive AI Readiness Model tailored for IT services companies, assessing readiness across four key pillars. Each pillar is critical for enabling generative AI to deliver real business impact. By assessing your organization against these dimensions, you can identify gaps and take actionable steps to become "AI-ready."
Readiness Pillar | Key Focus Areas & Criteria |
---|---|
Data Maturity | Data quality, accuracy and consistency; Robust data governance (security, privacy compliance); Sufficient volume & diversity of data for training; Accessible data infrastructure (warehouses, lakes) for AI use; Single source of truth to avoid silos. |
Technical Infrastructure | Scalable computing resources (cloud, GPU clusters) for AI workloads; AI platforms and tools (ML frameworks, APIs) in place; Data pipelines and integration architecture to feed AI models; MLOps capabilities for model development, deployment and monitoring; Reliability and performance to support AI in production. |
Skills & Talent Readiness | In-house AI expertise (data scientists, ML engineers, NLP specialists); Training and upskilling programs for staff (AI literacy, prompt engineering, data analysis); Hiring or partnering to fill skill gaps; Culture of innovation and learning (employees empowered to experiment with AI); Change management to support workforce adaptation. |
Operational Preparedness | Strategic alignment of AI projects with business goals and ROI; Executive leadership support and governance structure for AI; Policies for ethical AI use, risk management and compliance (responsible AI guidelines, security/privacy controls); Processes to integrate AI into workflows (from pilot to scale); Monitoring and metrics to track AI's impact. Without this foundation, AI projects often remain stuck in perpetual pilot mode, never scaling to deliver business impact. |
Data Maturity
The foundation for AI success
Data is the fuel of AI. This pillar assesses your organization's ability to manage, govern, and utilize data effectively, ensuring it's high-quality, accessible, and compliant.
Technical Infrastructure
Scalable tools and platforms for AI
The right tech architecture, hardware, software, and integration capabilities needed to develop, deploy, and scale AI solutions effectively and reliably.
Skills & Talent
Building an AI-capable workforce
The human element: having the right expertise to develop, implement, and use AI, from technical specialists to AI-literate employees throughout the organization.
Operational Preparedness
Strategy, processes & governance for AI
The strategic and managerial aspects: leadership alignment, processes for integrating AI, and frameworks for governing AI use ethically and effectively.
Data Maturity
The foundation of AI success: quality data, governance, and accessibility
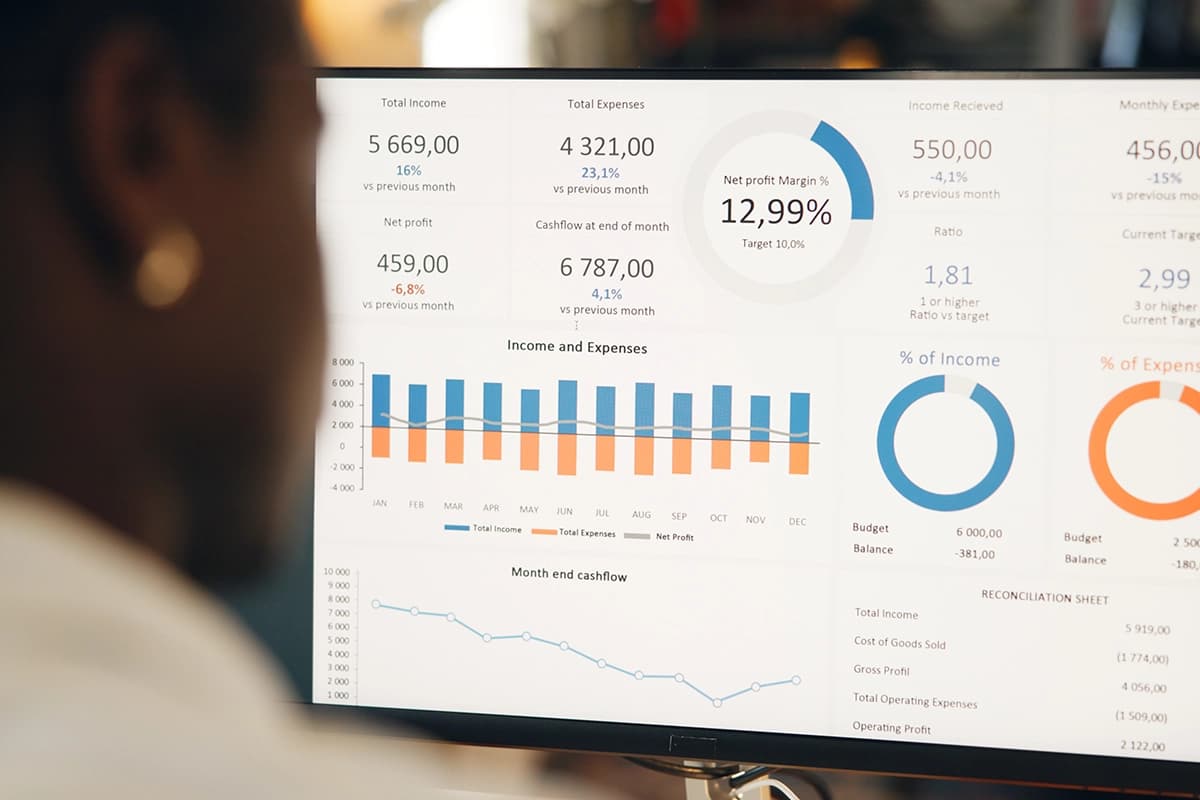
The Critical Foundation
Data Maturity is the foundational pillar of AI readiness. AI systems – particularly generative AI – are only as good as the data they learn from. This pillar assesses whether your organization has data of sufficient quality, quantity, and accessibility to power effective AI initiatives.
Without high-quality, well-governed data, even the most sophisticated AI models will produce poor results. The adage "garbage in, garbage out" is especially relevant for AI systems, which can amplify data flaws.
The Data Challenge
Research shows that 43% of organizations cite data quality and availability as their biggest barrier to AI adoption. For generative AI specifically, having diverse, well-structured, and contextually rich data is essential for models to generate accurate and valuable outputs.
A mature data organization doesn't just collect data – it treats data as a strategic asset, with clear governance, quality standards, and accessibility for those who need it, when they need it.
Technical Infrastructure
Building the technological backbone to develop, deploy, and scale AI
The Technological Foundation
Technical Infrastructure refers to the hardware, software, and architectural components needed to support AI workloads effectively. This pillar assesses whether your organization has the right tech stack to develop, deploy, and scale AI solutions.
Without adequate technical infrastructure, AI projects face bottlenecks in development, challenges in deployment, and limitations in scaling. The right infrastructure allows for efficient model training, seamless deployment, and reliable production performance.
The Infrastructure Gap
Many organizations underestimate the technical requirements for effective AI implementation. A 2023 survey found that 67% of companies had to significantly upgrade their infrastructure mid-project to support AI initiatives, leading to delays and cost overruns. Modern generative AI applications require especially robust infrastructure for real-time inference.
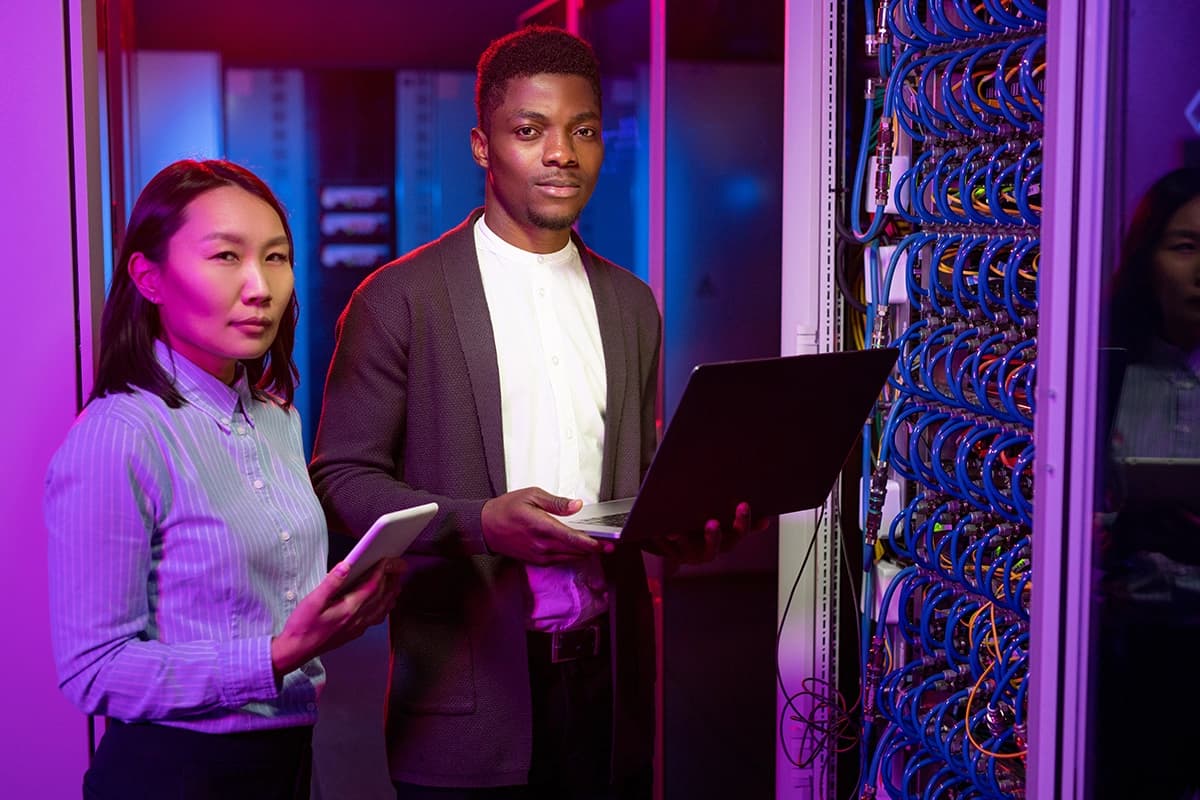
Skills & Talent Readiness
Building an AI-capable workforce is essential for sustainable AI success
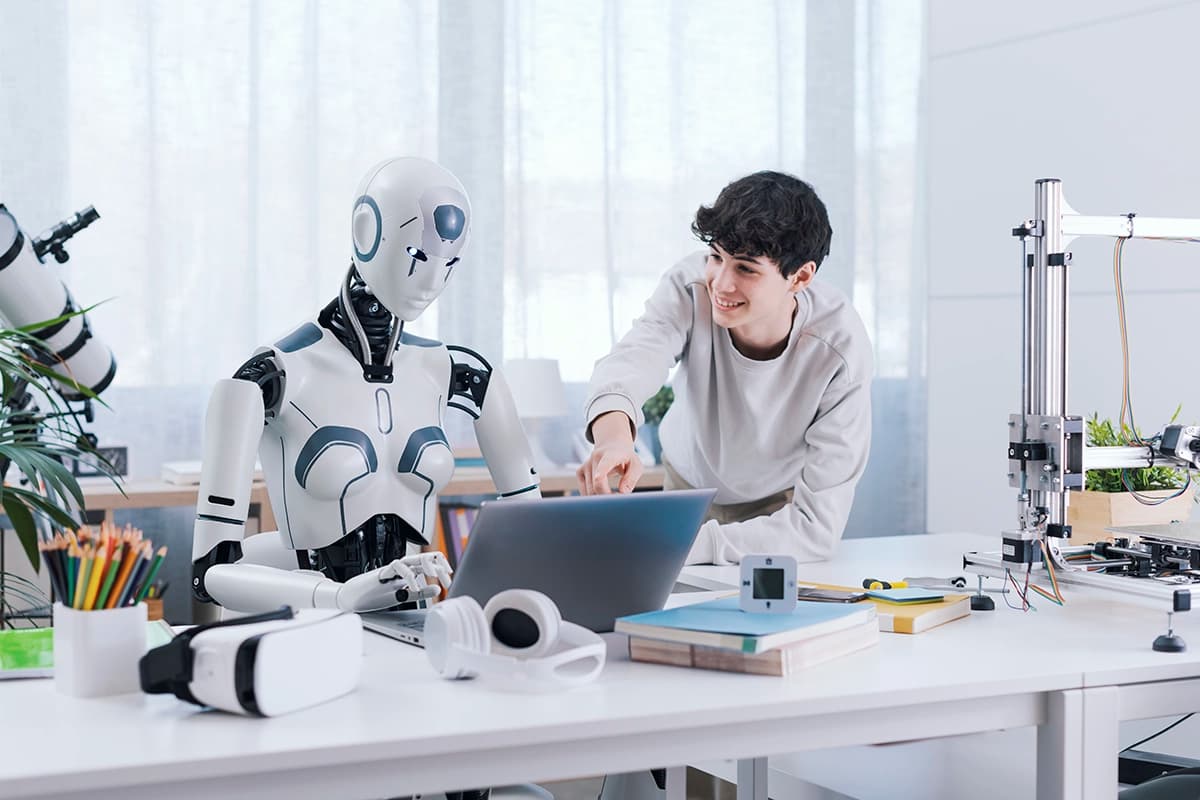
The Human Element of AI Success
Even with great data and tools, AI readiness ultimately depends on people. Skills & Talent Readiness refers to the human capital aspect: does the organization have the right expertise to develop, implement, and use generative AI?
This includes technical experts (data scientists, machine learning engineers, NLP specialists), but also extends to a broader base of employees who need sufficient AI literacy to work alongside AI systems or integrate them into business processes.
The Skills Challenge
Current research indicates a significant skills gap is a barrier to AI adoption. Globally, a shortage of skills and data literacy was identified by 35% of organizations as a top obstacle to AI project success. In Canada, despite our strong AI research community, many companies struggle to hire or develop the right talent for applied AI.
Operational Preparedness
Strategy, processes, and governance for successful AI integration
Strategy and Management for AI Success
The fourth pillar, Operational Preparedness, encompasses the strategic and managerial aspects of AI adoption. It asks whether the organization's leadership, processes, and risk frameworks are prepared to integrate AI into the business.
Without clear strategic alignment and operational integration, even technically sound AI projects fail to deliver value. This is the pillar that distinguishes AI leaders from laggards, ensuring that AI becomes part of your organization's DNA rather than a siloed experiment.
The Operational Gap
Many Canadian organizations lag behind global peers in integrating AI into their strategies and operations, leading to fewer realized benefits than expected. However, the same leaders recognize that organization-wide integration and transparent change management are key to building trust and unlocking productivity.
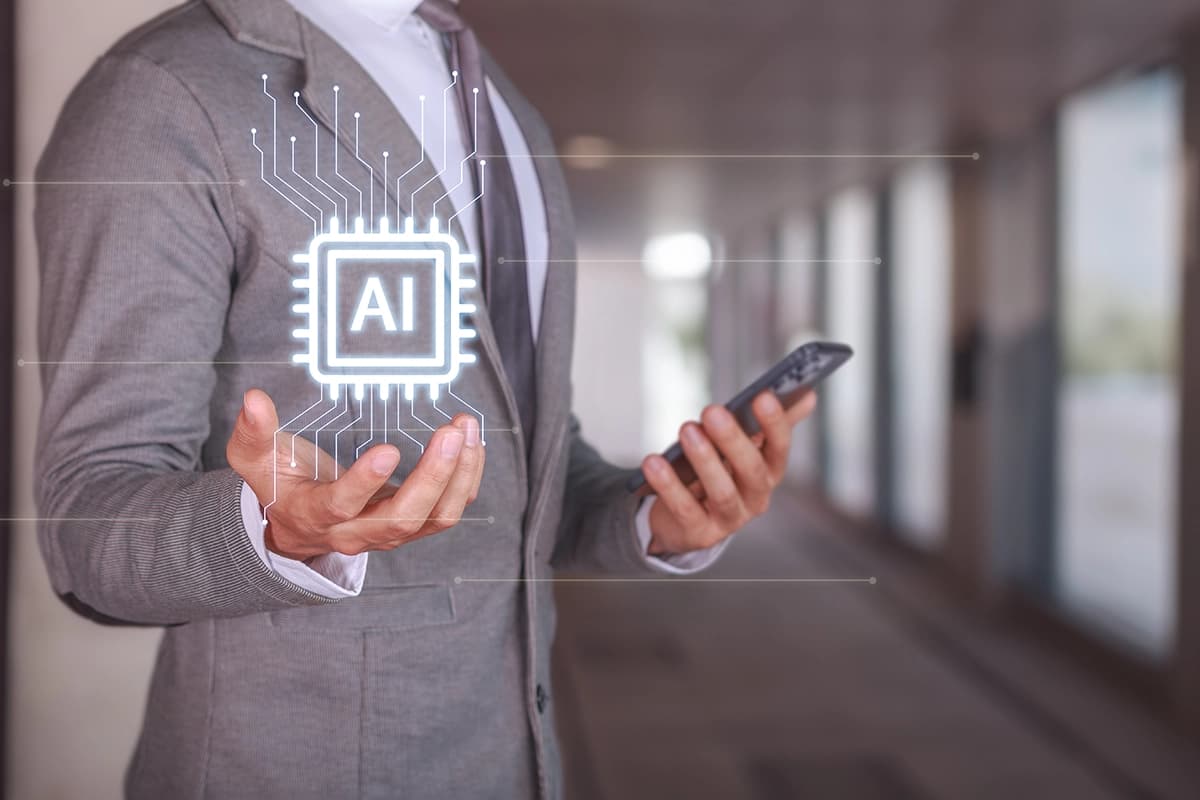
AI Readiness Self-Assessment
Evaluate your organization's readiness across the four key pillars
Get a Comprehensive Assessment
For a more detailed evaluation of your AI readiness with personalized recommendations, our team of experts can help with a thorough assessment and strategic roadmap.
Request Expert AssessmentWhat You'll Receive
In-depth AI Readiness Score
Detailed Gap Analysis
Custom AI Strategy Roadmap
Implementation Guidance
Frequently Asked Questions
Common questions about the AI Readiness Framework
Why is AI readiness important for IT services companies?
AI readiness is critical for IT services companies because it determines your ability to successfully implement and benefit from AI technologies. Without proper readiness across the four pillars (data, technical, skills, and operational), AI projects often fail to deliver expected value or scale beyond pilot stages. Given that over 80% of AI projects fail to deliver value, proper preparation across these dimensions significantly increases your chances of success and competitive advantage.
How long does it typically take to become AI-ready?
The timeframe to achieve AI readiness varies depending on your starting point and organizational size. Generally, organizations can make significant progress in 6-12 months with focused effort. Some dimensions may be addressed relatively quickly (like establishing an AI strategy or implementing AI tools), while others (like improving data maturity or building skills) may take longer. Rather than viewing readiness as a binary state, consider it as a continuous journey of improvement across all four pillars.
Which pillar should we prioritize first?
While all four pillars are important, most organizations should start with Data Maturity as a foundation, since AI is only as good as the data it learns from. Without quality data that's accessible and well-governed, even the best AI tools and talent will struggle to produce good results. That said, the best starting point depends on your specific situation. If you already have strong data practices but lack AI skills, then focusing on talent development might be more urgent.
Have other questions about implementing the AI Readiness Framework?
Talk to Our AI Readiness Experts